The ICDSST 2025 Conference is proud to feature invited speakers who will share their expertise on key topics in decision support systems.
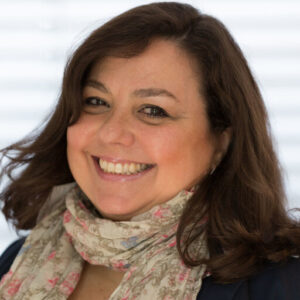
Fátima Dargam
SimTech Simulation Technology & REACH Innovation, Austria
CEO & Research Managing Director / Co-founder
Honorary Chair and Invited Speaker
Dr. Fátima Dargam is an expert in Decision Support Systems and Artificial Intelligence, with a Ph.D. from Imperial College London and extensive experience as an R&D IT Manager at SimTech in Austria. Her work spans intelligent decision-making, multi-agent systems, and big data analytics, with significant contributions through research, publications, and her role as a founding member and former coordinator of the EURO Working Group on DSS.
Title: Tracing the Evolution of AI Integration in Decision Support: A Review of EWG-DSS and ICDSST Contributions
Abstract:
Over the past three decades, Decision Support Systems (DSS) have increasingly incorporated Artificial Intelligence (AI) to enhance Decision-Making (DM) processes. The EURO Working Group on Decision Support Systems (EWG-DSS) has been at the forefront of this evolution. Already in the EWG-DSS foundation, at the EURO Summer Institute on DSS in the Madeira Island in1989, an Expert System that instantiated the way that AI could be used in DSS for military applications was presented. Following that, the EWG-DSS has promoted a rich body of research, through its annual organized Workshops and the International Conferences on DSS Technology (ICDSST), capturing how AI techniques have become integral to modern DSS. The current Abstract-Review synthesizes key insights from EWG-DSS and ICDSSTs published contributions, including Workshops and Conferences Proceedings; Springer LNBIP volumes; and Journal Special Issues, to trace the historical evolution of AI in DSS within our scenario. This abstract also highlights major technical and methodological innovations, identifying emerging thematic trends, like: Cognitive DSS, Sustainability, Data Visualization, Collaborative Decision-Making, among others; and emphasizing the role and importance of international and multidisciplinary research collaboration in those developments.
Overall, the progression of the usage of AI-based approached reviewed illustrates a clear trajectory from early experiments in AI-driven decision support to today’s sophisticated AI-rich DSS paradigms, most recently with the integration of Generative AI to support decision making in various applications.
The evolution of DSS, as traced through the perspective of the EWG-DSS and the ICDSST contributions, showcases a dynamic interplay between Decision Science and AI Technology. From early knowledge-based systems to today’s cognitive and data-driven decision platforms, AI integration has continually expanded the capabilities and scope of DSS. Notably, this progress has been driven by robust international collaboration and multidisciplinary efforts. The EWG-DSS network itself, grown from a small 1989 gathering to over 350 members worldwide, exemplifies how diverse expertise (spanning operational research, computer science, and domain specialties) has merged to push DSS research forward. Such collaboration has yielded significant contributions / publications; and has also fostered innovation in methodologies and applications of DSS. Hence, the past thirty years of the EWG-DSS work reveal a field that has continually reinvented itself by embracing AI advancements and new decision challenges. This ongoing synergy between AI and DSS promises to further enhance decision support technologies for complex problem-solving in the years ahead.
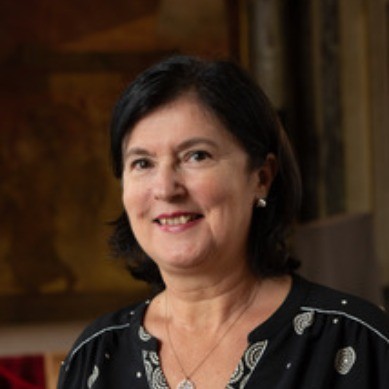
Pascale Zaraté
Université Toulouse Capitole- IRIT
Full Professor
Honorary Chair and Invited Speaker
Pascale Zaraté is a professor at Toulouse Capitole University, France. She conducts her research at the IRIT Laboratory and chair the Artificial Intelligence department. She holds a PhD. at University Paris Dauphine, Paris (1991) and a Habilitation to Conduct Research at Institut national Polytechnic Toulouse. Pascale Zaraté’s current research interests include: decision support systems, cooperative decision-making, Group Decision Making. She has been coordinator of the European working group on DSS for 20 years. She is currently President of the INFORMS GDN section. She edited more than 30 special issues in several international journals, several books and proceedings of international conferences. She has published 3 books and more than 40 articles in several international journals, seven chapters in collective works, a more than 60 articles in international conferences. She is a member of the scientific editorial board of seven international journals. She organised several international conferences focusing on Decision Support Systems.
Title :
Decision Support: History and trends
Abstract:
Decision Support Systems (DSS) were born in the 70’s. Since this decade, we can find in the literature several tracks of evolution for these systems. We will draw the evolution of DSS: describing the architecture, the usability, the functionalities, the programming techniques as well as the way to use these systems. We will show how Artificial Intelligence and Machine Learning influenced the development of DSSs. Different projects will be presented related to DSSs.
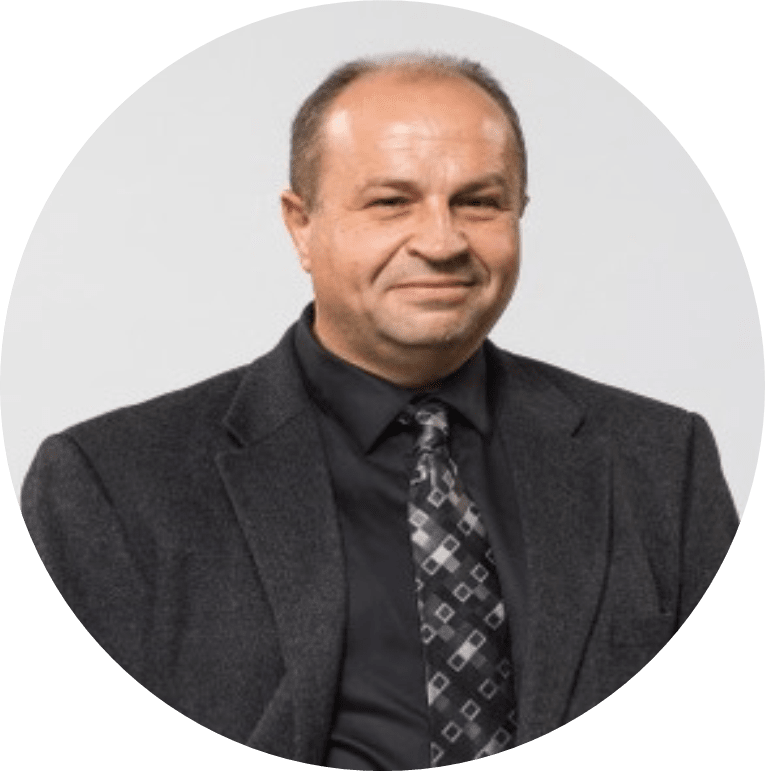
Zoran Obradović
Director, Center for Data Analytics and Biomedical Informatics
Temple University
Invited Speaker
Zoran Obradovic is a Distinguished Professor and a Center director at Temple University, an Academician at the Academia Europaea (the Academy of Europe), and a Foreign Academician at the Serbian Academy of Sciences and Arts. He mentored about 50 postdoctoral fellows and Ph.D. students, many of whom have independent research careers at academic institutions (e.g. Northeastern Univ., Ohio State Univ,) and industrial research labs (e.g. Amazon, eBay, Facebook, Hitachi Big Data, IBM T.J.Watson, Microsoft, Yahoo Labs, Uber, Verizon Big Data, Spotify). Zoran is the editor-in-chief of the Big Data journal and the steering committee chair for the SIAM Data Mining conference. He is also an editorial board member of 13 journals and was the general chair, program chair, or track chair for 11 international conferences. His research interests include data science and complex networks in decision support systems addressing challenges related to big, heterogeneous, spatial, and temporal data analytics motivated by applications in healthcare management, power systems, earth, and social sciences. His studies were funded by AFRL, DARPA, DOE, NIH, NSF, ONR, PA Department of Health, US Army ERDC, US Army Research Labs, and industry. He published about 450 articles and is cited more than 34,000 times (H-index 70). For more details see: http://www.dabi.temple.edu/zoran-obradovic
Talk:
Lifting the Fog by Harnessing Interactions Across Diverse Systems
Abstract:
This presentation will discuss our machine learning approaches for identifying, categorizing, and forecasting events of interest from limited data and imprecise labels. This is accomplished by integrating multimodal structured and unstructured information and jointly modeling multiple types of interactions in temporal multilayer networks. Our findings indicate that a learning framework that integrates information from various sources of differing quality and resolution can significantly enhance informed decision-making.
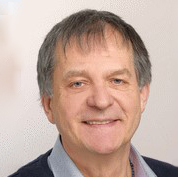
Marko Bohanec
Scientific Councillor
Department for Knowledge Tehnologies, Jožef Stefan Institute.
Marko Bohanec received a Ph.D. in Computer Science from the University of Ljubljana, Slovenia, in 1991. He is a leading Slovenian researcher in decision support models and systems. He currently serves as a Scientific Councillor at the Department of Knowledge Technologies, Jožef Stefan Institute, and a Full Professor of Computer Science at the University of Nova Gorica, Slovenia. His research interests include decision making, decision analysis, decision support, decision modeling, artificial intelligence, expert systems, machine learning, and data mining. He is a co-author of the method DEX (Decision EXpert) and its associated software, widely used for qualitative hierarchical multi-attribute decision modeling. Over the past decade, he has contributed as a decision support expert and developer of decision support systems in European projects spanning food and feed production, distribution, and quality control; healthcare disease management; nuclear safety; and sustainable mobility.
Talk:
Exploring Synergies Between Human and Artificial Intelligence:
The Case of the DEX Decision Modeling Method
Abstract:
Knowledge-driven Decision Support Systems (DSS) rely primarily on expert knowledge, rules, and heuristics to analyze situations and provide recommendations. Traditionally, they have been developed through Expert Modeling (EM), the process of capturing, formalizing, and structuring human expertise. However, the rise of Machine Learning (ML) has often overshadowed EM, creating the illusion that complex decision-making can be reduced to simply “pressing a button” to generate models from data. While ML is considered modern and efficient, critical requirements such as correctness, completeness, consistency, comprehensibility, and convenience often necessitate the integration of EM. This lecture explores the synergy between ML and EM, emphasizing the importance of combining AI with human expertise to develop robust DSS. We will examine how Large Language Models (LLMs) can assist in structuring decision criteria and modeling preferences, while acknowledging their limitations in capturing human decision preferences. These aspects will be illustrated using the qualitative decision modeling method DEX (Decision EXpert).
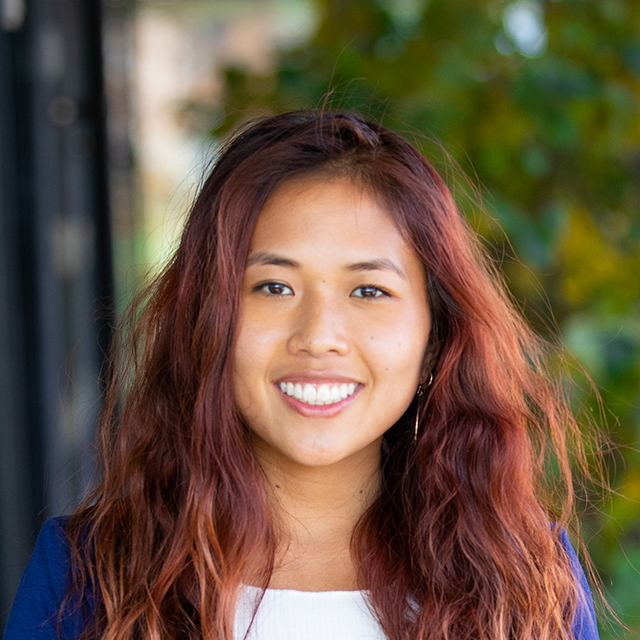
Lily Xu
Postdoc at Oxford, (soon Assistant Professor at Columbia)
Invited Speaker
Lily Xu develops methods across machine learning, optimization, and causal inference for planetary health challenges, with a focus on biodiversity conservation. She aims to enable practitioners to make effective decisions in the face of limited data, taking actions that are robust to uncertainty, effective at scale, and future-looking. In her work, Lily partners closely with NGOs to bridge research and practice, serving as AI Lead for the SMART Partnership. Since 2020, she has co-organized the EAAMO research initiative, committed to advancing Equity and Access in Algorithms, Mechanisms, and Optimization. Lily will join Columbia IEOR as an Assistant Professor in July 2025.
Talk:
High-stakes decisions from low-quality data: AI decision-making for planetary health
Abstract:
Planetary health recognizes the inextricable link between human health and the health of our planet. Our planet’s growing crises include biodiversity loss, with animal population sizes declining by an average of 70% since 1970, and maternal mortality, with 1 in 49 girls in low-income countries dying from complications in pregnancy or birth. Overcoming these crises will require effectively allocating and managing our limited resources. My research develops data-driven AI decision-making methods to do so, overcoming the messy data ubiquitous in these settings. Here, I’ll present technical advances in multi-armed bandits, robust reinforcement learning, and causal inference, addressing research questions that emerged from on-the-ground challenges across conservation and maternal health. I’ll also discuss bridging the gap from research and practice, with anti-poaching field tests in Cambodia, field visits in Belize and Uganda, and large-scale deployment with SMART conservation software.